Spatial and temporal Characterization of Forecasting GW Anomalies in Wisconsin Central Sands (WCS)
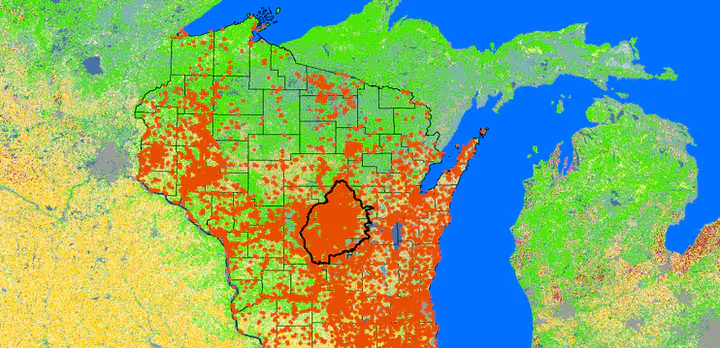
Wisconsin is humid, though GW shortages have been reported in several regions, including the Wisconsin Central Sands (WCS). The shortage of GW in the WCS is caused by many factors, including climate variability, fast expansion of irrigation, increasing high-capacity wells, and increasing population. For sustainable water resource management, there is an urgent need to develop a computationally-efficient and easy-to-update framework with the capability of efficient incorporation of recently developed and regularly updated environmental datasets (e.g., remote sensing maps of evapotranspiration and soil moisture).
The research questions for this project include: Using computationally efficient ML models, can easy-to-obtain remote sensing derived environmental datasets be combined to accurately predict GW dynamics across the WCS? How does GW anomaly vary in space and across the WCS? How pumping and crop type change can influence this anomaly forecasting?
Static and dynamic variables were used to build LSTM model for prediction of GW depth at the 26 well locations in the WCS region monthly from 1958 to 2020.
Based on the findings, overall palmer drought severity index (PDSI) and soil moisture has high correlation with GW elevation. Other Important predictor include previous 5-month Tmax and solar radiations.
Two-month GW anomaly forecast temporal model can explain variance 90% in Northern region. Model MAE is higher when previous month GWE has lower autocorrelation.
In our observations, negative anomaly means shallow GW, so pumping has greater influence on shallow GW. Hence model can better estimate anomaly for shallower water (Figure 6). Pumping makes GW deeper so the anomaly becomes positive. I hope to submit my manuscript for this research project by January 2022.